Table Of Content
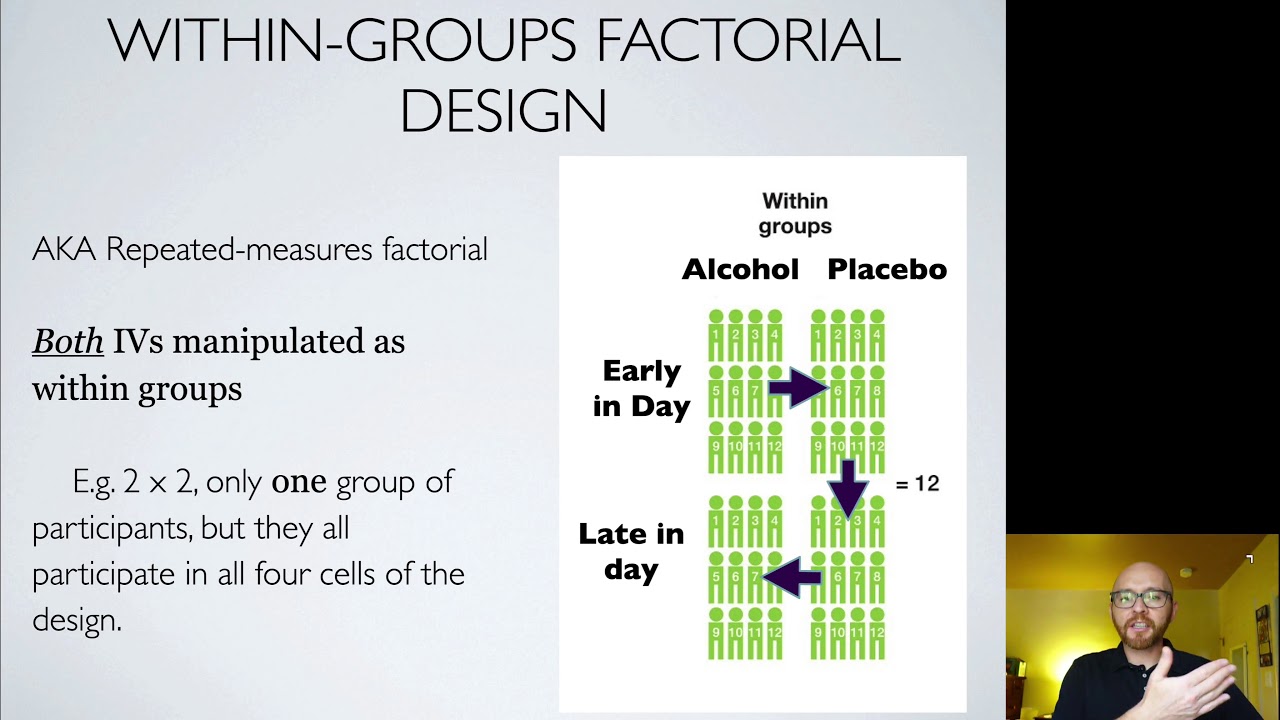
This might result in subadditive or negative interactions in which interventions produce less benefit, or even produce net decreases in benefit, when they co-occur with another intervention(s). This can pose interpretive challenges as it may be difficult to separate the effects of a component per se from the impact of burden. If an investigator anticipates severe problems from including a particular factor in an experiment, perhaps due to its nature or the burden entailed, s/he should certainly consider dropping it as an experimental factor. Indeed, the MOST approach to the use of factorial designs holds that such designs be used to decompose a set of compatible ICs, ones that might all fit well in an integrated treatment package (to identify those that are most promising). That is, one should include only those ICs that are thought to be compatible, not competitive. In an RCT an “active” treatment arm or condition is statistically contrasted with a “control” treatment arm or condition (Friedman, Furberg, & Demets, 2010).
Implementing Clinical Research Using Factorial Designs: A Primer
These independent variables are good examples of variables that are truly independent from one another. For example, shoes with a 1 inch sole will always add 1 inch to a person’s height. This will be true no matter whether they wear a hat or not, and no matter how tall the hat is. In other words, the effect of wearing a shoe does not depend on wearing a hat.
How to Deal with a 2n Factorial Design

There are no inferences obtained and therefore cannot be generalized to the population which is a limitation. Most often than not, a series of case reports make a case series which is an atypical presentation found in a group of patients. This in turn poses the question for a new disease entity and further queries the investigator to look into mechanistic investigative opportunities to further explore.
1.4. Measures of the Same Construct¶
One independent variable was disgust, which the researchers manipulated by testing participants in a clean room or a messy room. The other was private body consciousness, a variable which the researchers simply measured. Another example is a study by Halle Brown and colleagues in which participants were exposed to several words that they were later asked to recall [BKD+99]. Some were negative, health-related words (e.g., tumor, coronary), and others were not health related (e.g., election, geometry). The non-manipulated independent variable was whether participants were high or low in hypochondriasis (excessive concern with ordinary bodily symptoms). Results from this study suggested that participants high in hypochondriasis were better than those low in hypochondriasis at recalling the health-related words, but that they were no better at recalling the non-health-related words.
Recall that in a between-subjects single factor design, each participant is tested in only one condition. It is also possible to manipulate one independent variable between subjects and another within subjects. The number of ICs may affect the clinical relevance and generalizability of the research findings. Increased numbers of ICs and assessments may create nonspecific or attentional effects that distort component effects. For instance, while a real world application of a treatment might involve the administration of only two bundled ICs (counseling + medication), a factorial experiment might involve 6 or more ICs.
This exercise will how the condition means are used to calculate the main effects and interactions. We have discussed various clinical research study designs in this comprehensive review. Though there are various designs available, one must consider various ethical aspects of the study.
However, factorial design can only give relative values, and to achieve actual numerical values the math becomes difficult, as regressions (which require minimizing a sum of values) need to be performed. Regardless, factorial design is a useful method to design experiments in both laboratory and industrial settings. In principle, factorial designs can include any number of independent variables with any number of levels. For example, an experiment could include the type of psychotherapy (cognitive vs. behavioral), the length of the psychotherapy (2 weeks vs. 2 months), and the sex of the psychotherapist (female vs. male). This would be a 2 x 2 x 2 factorial design and would have eight conditions. In practice, it is unusual for there to be more than three independent variables with more than two or three levels each.
Achieving the Right Component Comparisons
This will allow you to determine the effects of temperature and pressure while saving money on performing unnecessary experiments. The following Yates algorithm table using the data from the first two graphs of the main effects section was constructed. Besides the first row in the table, the row with the largest main total factorial effect is the B row, while the main total effect for A is 0. This means that dosage (factor B) affects the percentage of seizures, while age (factor A) has no effect, which is also what was seen graphically.
Factorial Experimental Design
However, we can also perform a two-way ANOVA to formally test whether or not the independent variables have a statistically significant relationship with the dependent variable. Plotting the means is a visualize way to inspect the effects that the independent variables have on the dependent variable. For example, imagine that a researcher wants to do an experiment looking at whether sleep deprivation hurts reaction times during a driving test. If she were only to perform the experiment using these variables–the sleep deprivation being the independent variable and the performance on the driving test being the dependent variable–it would be an example of a simple experiment.
Design of Experiments with R. Building 2^k Factorial Designs by Roberto Salazar - Towards Data Science
Design of Experiments with R. Building 2^k Factorial Designs by Roberto Salazar.
Posted: Tue, 03 Dec 2019 05:32:29 GMT [source]
That is, the levels of each independent variable are each manipulated across the levels of the other indpendent variable. In other words, we manipulate whether switch #1 is up or down when switch #2 is up, and when switch numebr #2 is down. A factorial trial study design is adopted when the researcher wishes to test two different drugs with independent effects on the same population. Typically, the population is divided into 4 groups, the first with drug A, the second with drug B, the third with drug A and B, and the fourth with neither drug A nor drug B.
Assessing Thermoelectric Membrane Distillation Performance: An Experimental Design Approach - ScienceDirect.com
Assessing Thermoelectric Membrane Distillation Performance: An Experimental Design Approach.
Posted: Tue, 06 Feb 2024 07:29:04 GMT [source]
When the independent variable is a construct that can only be manipulated indirectly—such as emotions and other internal states—an additional measure of that independent variable is often included as a manipulation check. This is done to confirm that the independent variable was, in fact, successfully manipulated. For example, Schnall and her colleagues had their participants rate their level of disgust to be sure that those in the messy room actually felt more disgusted than those in the clean room. In this situation, the investigators were uninterested in the effects of behavioral therapy alone. Nonetheless, the conducted studies confounded several design features that slightly complicate the interpretation of their findings.
But if it showed that you did not successfully manipulate participants’ moods, then it would appear that you need a more effective manipulation to answer your research question. Again, because neither independent variable in this example was manipulated, it is a cross-sectional study rather than an experiment. In the first study, participants were randomly assigned to receive either 3 weeks of light flashes (light alone) or a sham light intervention. The light flashes were brief light pulses administered in 3-millisecond bursts delivered 20 seconds apart, starting 3 hours before the targeted wake time for the individual participant. In the second study, participants were randomized to a combination of light plus cognitive behavioral therapy or sham light therapy plus cognitive behavioral therapy. The main outcomes were self-reported sleep times, momentary ratings of evening sleepiness, and subjective measures of sleepiness and sleep quality.
This fails to prove if the outcome was truly due to the intervention implemented or due to chance. This can be avoided if a controlled study design is chosen which includes a group that does not receive the intervention (control group) and a group that receives the intervention (intervention/experiment group), and therefore provide a more accurate and valid conclusion. Cohort studies are typically chosen as a study design when the suspected exposure is known and rare, and the incidence of disease/outcome in the exposure group is suspected to be high. The choice between prospective and retrospective cohort study design would depend on the accuracy and reliability of the past records regarding the exposure/risk factor.
A factorial design is a type of experiment that involves manipulating two or more variables. While simple psychology experiments look at how one independent variable affects one dependent variable, researchers often want to know more about the effects of multiple independent variables. Consistently, it has been suggested that properly conducted factorial trials may be the best available way to investigate whether an interaction exists between treatments (McAlister et al. Reference McAlister, Straus, Sackett and Altman2003). Most complex correlational research, however, does not fit neatly into a factorial design. Instead, it involves measuring several variables, often both categorical and quantitative, and then assessing the statistical relationships among them. For example, researchers Nathan Radcliffe and William Klein studied a sample of middle-aged adults to see how their level of optimism (measured by using a short questionnaire called the Life Orientation Test) was related to several other heart-health-related variables [RK02].
No comments:
Post a Comment